Machine learning (ML) is becoming integral to fintech innovation. Nowadays, it is powering everything from fraud detection to customer service chatbots. However, implementing these ML solutions comes at a cost – often a significant one. In fact, adopting AI can be especially expensive for smaller fintech startups, and many struggle with the high costs involved. Understanding what drives these expenses is crucial for fintech entrepreneurs and investors. This article breaks down the key factors influencing ML development costs in fintech, compares the cost implications of different AI technologies, and illustrates these points with real-world insights and a case study.
Key Factors Influencing ML Development Costs in Fintech
Several factors determine how much a fintech company will spend on developing and deploying a machine learning solution. By examining these factors – and how they vary by use case – fintech leaders can better estimate budgets and find cost-effective strategies. Below are the major cost drivers for ML projects in fintech, each accompanied by examples of their impact:
- Data Volume and Quality: ML thrives on data. Large, high-quality datasets often need to train accurate models, especially in fintech applications like fraud detection that analyze millions of transactions. Acquiring and preparing this data can be costly and time-consuming. For example, a fraud detection system may require years of transaction data and synthetic data generation to cover edge cases, whereas a simple chatbot might rely on a smaller set of conversational scripts. More data also means higher storage and processing costs.
- Infrastructure and Technology Stack: The choice of infrastructure – cloud vs. on-premise, and the tech stack – can greatly affect cost. Real-time, high-throughput use cases might need expensive, scalable cloud computing or even specialized hardware for low-latency processing. In contrast, a basic predictive analytics model might run on standard cloud servers. Opting for cloud services can offer flexibility and lower upfront costs, while on-premises deployments entail heavy investments in hardware, security, and maintenance. Many fraud systems use big-data frameworks and ML libraries, which can increase costs for licensing and engineers to manage them.
- Model Complexity and ML Technique: The complexity of the AI solution is a major cost determinant. A simple rule-based or logistic regression model is cheaper to develop than a sophisticated deep learning or graph neural network model. For instance, a basic rule-based chatbot might be built for under $15k, while an advanced AI chatbot with natural language processing can cost well into six figures. Similarly, a fraud detection AI that continuously learns from new patterns will be more complex than a static credit-scoring model. Complex models also take longer to develop and require more computational power for training.
- Regulatory Compliance & Security: Fintech is heavily regulated, requiring compliance with standards like GDPR (data privacy), PCI-DSS (payment security), and AML/KYC (anti-fraud). Ensuring compliance demands extra development for data anonymization, audit trails, explainability, fairness, and thorough security testing. These safeguards are critical, as breaches or violations can result in costly fines and reputational damage. Incorporating robust compliance and security typically adds tens of thousands of dollars to fintech ML project costs.
- Talent & Development Team: Building fintech ML solutions requires skilled data scientists, ML engineers, and domain experts, who command high salaries due to strong market demand. Annual salaries for data scientists typically range from $80k–$200k, and ML engineers from $50k–$150k. Complex projects may also involve domain specialists (e.g., fraud analysts) and integration developers. Choosing between an in-house team (ongoing salary and benefits) or outsourcing (potentially lower initial costs but added communication overhead) impacts expenses. High-quality talent significantly influences project success and overall costs.
- MLOps & Ongoing Maintenance: Launching an ML model involves continuous maintenance costs, including performance monitoring, detecting data drift, and periodic retraining. This practice, known as MLOps, ensures models stay accurate as data and business conditions evolve. Industry standards recommend budgeting roughly 15–20% of initial development costs annually for maintenance, with even higher expenses possible in fast-changing environments. Neglecting ongoing upkeep can result in costly model failures, poor decisions, or security risks.
In summary, high-complexity, mission-critical ML applications will incur costs on all these fronts – large data pipelines, robust infrastructure, top talent, strict compliance checks, and intensive ongoing monitoring. Simpler use cases hit fewer cost drivers and can be developed for a fraction of the price. As one fintech development report notes, a basic fintech app might cost under $50k, whereas a complex platform with AI features can cost $150k–$500k+ to build.
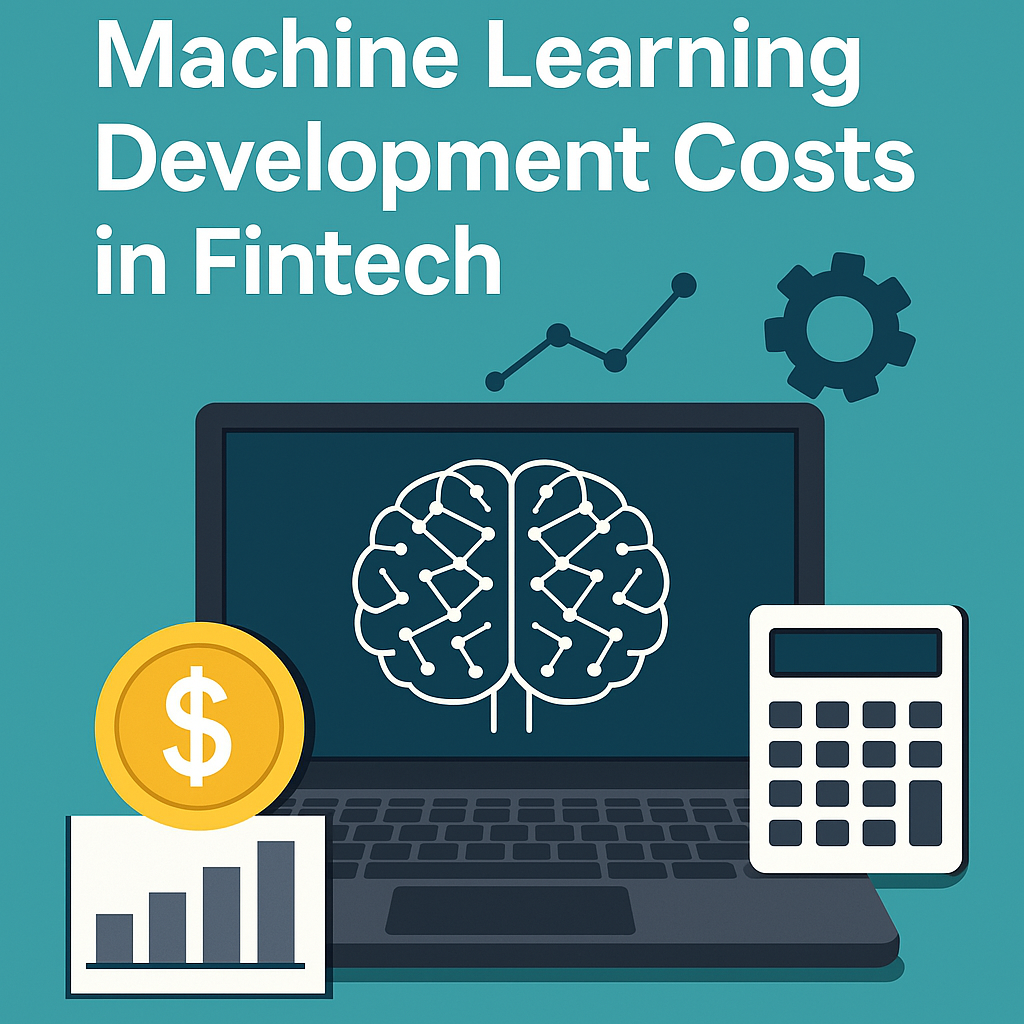
Business Advantages of ML in Fintech (and ROI Implications)
Despite the high development costs, machine learning offers compelling business advantages for fintech companies – advantages that can translate into cost savings or revenue gains, providing a return on investment (ROI) for the ML project. It’s important to view ML spending not just as a cost center but as an investment that, if executed well, can pay for itself through efficiencies and improved outcomes. Below we highlight key benefits of ML in fintech and tie each to cost or ROI considerations relevant to fintech entrepreneurs:
- Enhanced Fraud Prevention & Cost Savings: AI-driven fraud detection significantly reduces financial losses by identifying fraudulent activities in real-time and minimizing false positives. By preventing fraud like credit card scams and money laundering, fintech companies directly save substantial sums. Industry reports estimate global savings from AI-based fraud detection will reach $10.4 billion by 2027, up 285% from $2.7 billion in 2022. Additionally, fewer false alarms reduce manual review efforts, lowering labor costs and improving operational efficiency. Despite initial investment, these systems rapidly deliver strong ROI by preventing fraud and optimizing resource allocation.
- Automation of Customer Service: AI-powered chatbots can significantly reduce customer service costs — by up to 80% — and enhance response accuracy, according to IBM estimates. For fintech startups, chatbots efficiently handle increased customer interactions without proportional staffing costs, enabling scalable growth. Although chatbot development incurs initial expenses, the savings in reduced headcount and operational efficiency quickly justify the investment. Additionally, chatbots enhance customer satisfaction through faster and more accurate support, driving long-term retention and ROI.
- Automation & Personalization for Revenue Growth: ML-driven credit scoring and robo-advisors help fintechs accurately assess risk and personalize offerings, enabling approval of more profitable loans and boosting customer acquisition. Improved loan conversions, reduced defaults, and personalized investment portfolios significantly increase revenue and market share. While sophisticated personalization (e.g., federated learning) may increase development complexity, the resulting revenue uplift typically justifies the investment. In short, advanced ML personalization strategies directly drive fintech growth and deliver attractive ROI.
- Operational Efficiency & Scalability: ML helps fintechs automate routine tasks like transaction categorization, document processing, and loan underwriting, enabling scalable growth without proportional cost increases. Automating previously manual tasks reduces errors, improves compliance, and accelerates operations—like rapidly processing loan applications or digitally verifying identities. AI-driven identity verification alone could save the industry hundreds of millions of dollars by 2028. Ultimately, ML automation allows fintech firms to scale efficiently, streamline workflows, and significantly cut operational costs.
- Risk Management & Compliance Efficiency: Machine learning significantly enhances fintech risk management by automating compliance monitoring (e.g., AML transaction checks) and proactively identifying risks. Although upfront costs are high, ML reduces regulatory fines and streamlines compliance staff workloads by prioritizing genuine threats over false alerts. Investors value ML-driven risk management, as it safeguards their investment, improves operational efficiency, and minimizes costly compliance failures.
Shortly speaking, the business benefits of ML in fintech strongly tie back to financial outcomes: either by cutting costs or by boosting revenue. For example, a $200k investment in a fraud detection model might prevent $500k in fraud losses in its first year, or a $50k AI chatbot might save $200k/year in customer service salaries – these are tangible ROI cases. Indeed, one AI provider reported their ML fraud solution reduced losses by 40%, which for a fintech could mean saving on the order of $10–50 million per year. The exact numbers will vary, but savvy fintech leaders will always map the costs of ML development to the potential financial upside. Doing so ensures that even if an ML project carries a hefty price tag, it is seen as a strategic investment with clear payback, rather than just a tech expense.
Case Study: AI-Powered Fraud Detection in a Fintech Startup
Company & Fraud Problem
FinSecure, a digital payments fintech startup, was facing rising fraud in its online transactions. The company was losing roughly $1 million annually to fraudulent activities, significantly impacting its bottom line. This escalating fraud problem highlighted the need for a more advanced fraud prevention solution to safeguard transactions and reduce losses.
AI Solution & Implementation Cost
FinSecure invested $150,000 in an AI-powered fraud detection system to combat these losses. The solution leverages supervised machine learning (ML) algorithms and anomaly detection techniques to identify suspicious transactions. Cloud-based ML models were integrated for real-time transaction scoring, providing instant risk assessments on each payment. The system also uses federated learning to continuously improve fraud pattern recognition across data sources, enhancing its effectiveness over time.
Outcomes & ROI
In the first year of using the AI system, FinSecure saw fraud losses drop by 40%, saving approximately $400,000 in prevented fraud. The automated detection also eliminated the need to hire three additional fraud analysts, avoiding about $200,000 in annual salary costs. In total, the company saved around $600,000 in the first year alone. This far exceeded the $150K implementation cost, so the solution essentially paid for itself within 12 months and delivered a strong return on investment (ROI).
Key Takeaways
- Rapid ROI: The ML-driven fraud detection system paid for itself within one year, demonstrating clear cost-effectiveness.
- Cost Savings: AI-based fraud detection reduced losses by 40% and saved FinSecure ~$600K in the first year, directly boosting the bottom line.
- Scalability: The solution offers long-term scalability, handling growing transaction volumes without significant new costs.
- Improved Compliance: Stronger fraud controls and real-time monitoring help meet regulatory compliance requirements and reduce risk.
For fintech startups, this case study highlights that ML investments can yield quick and substantial returns if targeted at the right problem. Fraud detection is an area where ROI is often quantifiable, which helps in making the business case to stakeholders. The same logic can apply to other areas like automating loan approvals, dynamic pricing models, or AI-driven customer acquisition strategies. The key is to measure and track the impact post-implementation, so you can truly see the cost/benefit balance.
Cost Implications of Key ML Technologies in Fintech
Not all machine learning use cases are equal when it comes to development cost. Different ML technologies and applications in fintech have varying cost profiles due to differences in complexity, required expertise, and infrastructure needs. Here we compare a few key AI solutions commonly used in fintech, shedding light on why some are more expensive than others:
Here’s the summarized cost profile in a clear, comparative table:
ML Use Case | Cost Profile | Typical Cost Range | Key Cost Factors | ROI / Benefits |
---|---|---|---|---|
Customer Service Chatbots & Virtual Assistants | Low to Moderate | $10k–$80k (up to $250k for advanced AI) | Integration, domain-specific refinement, maintenance | Cost savings, improved customer service |
Fraud Detection & Security Systems | High | $80k–$200k+ (over $1M for large banks) | Real-time data, advanced algorithms, regulatory compliance, continuous model updates, infrastructure demands | Significant reduction in fraud losses, high ROI |
Credit Scoring & Risk Modeling | Moderate to High | Tens to hundreds of thousands ($100k+) | Compliance & fairness testing, explainability, data acquisition, interface design | Improved lending decisions, reduced defaults, revenue growth |
Personalized Financial Advisory (Robo-Advisors & Recommendation Engines) | Moderate | Tens of thousands; increases with complexity | UX design, user trust/transparency, compliance, advanced NLP interactions | Enhanced engagement, retention, indirect revenue increase |
(Aside from the above, fintech also sees ML applied in areas like algorithmic trading, marketing analytics, and more – each with their own cost quirks. For example, high-frequency trading algorithms may resemble fraud detection in their need for speed and complexity, whereas marketing ML might be closer to the recommendation engines in cost. The key is to evaluate each ML use case on its demands for data, complexity, compliance, and required accuracy.)
To directly address a common question: Why is a fraud detection AI system so much more expensive to build than, say, a chatbot? As we’ve outlined, fraud AI demands heavier infrastructure, more complex modeling, continuous updates, and has higher stakes. A chatbot, while not trivial, can often be built on existing models and doesn’t require the same level of rigorous real-time data crunching or regulatory overhead. In numerical terms, you might build a functional AI chatbot for maybe $50k, whereas a robust custom fraud detection platform could easily cost 5–10 times that, as reflected in typical budgets. Knowing these differences in cost drivers can help fintech leaders prioritize which AI projects to tackle first and how to allocate resources.
Actionable Takeaways for Fintech Leaders
Managing machine learning development costs in fintech is a balancing act between innovation and budget discipline. Below are some practical tips for fintech entrepreneurs and investors to ensure ML projects deliver value for their cost:
- Align ML Projects with Clear Business ROI: Before diving into development, identify the specific pain point or opportunity the ML solution addresses – and quantify it. Whether it’s dollars saved from fraud prevention or new revenue from better customer insights, having a clear ROI goal helps justify costs and guide project scope. Focus on use cases with high impact-to-cost ratio (e.g., automating a costly manual process or preventing significant losses) as your first ML projects.
- Start Small with a Pilot (MVP): Rather than funding a massive, all-encompassing AI platform upfront, start with a Minimum Viable Product. For example, deploy an ML model for one segment of data or a simpler version of the problem, and test its effectiveness. This controlled approach keeps initial costs down and lets you validate the idea in a real-world setting. Early wins can secure buy-in for further investment, while early failures (on a small scale) provide learning without having sunk a fortune. Fintech leaders should iterate – improve the model gradually, add features over time – which spreads out costs and ensures you’re investing based on evidence of value.
- Leveraging Existing Solutions: Instead of building from scratch, fintech companies can save significantly by using pre-built cloud AI services, open-source frameworks, or pre-trained ML models. Many providers offer affordable APIs for common needs (NLP, anomaly detection, fraud detection). By strategically assembling these existing solutions, fintechs can cut development costs, accelerate implementation, and quickly achieve business goals.
- Invest in MLOps and Monitoring Early: It’s tempting to put off “maintenance” considerations during development, but in ML, a model without a maintenance plan will incur hidden costs later (or, worse, fail catastrophically). Implement basic MLOps early — monitor model performance, detect data drift, and schedule regular reviews — to avoid costly issues or model failure. Without ongoing maintenance, ML models can quickly degrade, leading to significant financial or operational losses. Plan to allocate around 10–20% of initial development costs annually for maintenance as a non-negotiable operational expense.
- Prioritize Data Quality & Security: Investing in high-quality, relevant data reduces development costs and enhances model performance. A focus on data quality shortens training cycles and minimizes debugging. Prioritizing security measures (anonymization, encryption, compliance with financial regulations) early prevents costly re-engineering and potential breaches, which can outweigh project costs entirely. Always budget for robust data compliance and security upfront.
- Tailor Complexity to the Problem: Choose the simplest ML solution that effectively addresses your fintech business needs. Overly complex models inflate costs without proportional benefits. Reserve advanced methods (deep learning, neural networks) for genuinely complex challenges like ID verification or sophisticated fraud detection. Using simpler models when possible prevents unnecessary expenses and avoids costly over-engineering.
- Understand and Communicate Trade-offs to Stakeholders: Fintech leaders should transparently explain ML project costs — breaking down expenses (data, infrastructure, talent, compliance) — and clearly outline expected benefits (e.g., reduced fraud losses, higher loan approvals, improved customer satisfaction). Providing clear metrics (fraud reduction, customer satisfaction, revenue impact) helps stakeholders understand value, ensures accountability, and keeps projects cost-effective over time.
- Plan for Gradual Scalability: Design fintech ML systems to scale from the outset to avoid costly re-engineering later. Adopt flexible cloud infrastructure that can easily handle future growth in users and transactions. However, scale resources incrementally to manage costs effectively. Leverage cost-saving practices, such as using spot instances or shutting down idle resources, to optimize expenses as you expand.
- Stay Current with Fintech ML Advances: The fintech ML landscape evolves quickly, with new tools, frameworks, and techniques regularly emerging that can significantly reduce development costs. Leveraging developments like AutoML or open-source fraud detection frameworks can accelerate prototyping and lower expenses. Stay informed and network actively—this can help identify cost-saving opportunities early, minimizing unnecessary effort and ensuring efficient investment.
Conclusion
In conclusion, managing machine learning development costs in fintech is about making strategic choices – what to build, how to build it, and how to maintain it – all grounded in a clear understanding of the value it will bring. Fintech leaders who navigate this well will find that ML isn’t just a cost center but a powerful driver of efficiency and growth. By starting smart, focusing on ROI, and remaining agile, you can harness the promise of AI in fintech without breaking the bank. The payoff, in terms of competitive advantage and financial returns, will make the effort worthwhile for both entrepreneurs and investors committed to the fintech space.