Today’s competitive environment is driving banks to turn away from product obsession, which erodes their margins, and focus on the customer by competing on the basis of customer experience. Everyone loves personal touch, that’s why it’s no longer enough to only offer the same products and services as your competitors. Modern customers believe that financial service providers offer necessary services that impact their future financial standing as individuals so they expect to get valuable insights and advice from their banks. Moreover, they expect them to be unique and individual.
Apart from the need to manage the growing expectations of their customers, banks face a host of other challenges, such as the threat of new entrants, changes forced by regulators, and the shift from face-to-face interaction to remote service and digital format.
Certainly, personalization is not the answer to everything, but it does help banks strengthen their position in the market and boost sales, which in turn leads to increased revenue. BCG, a global consulting firm, estimates that for every $100 billion in assets, a bank can achieve up to $300 million in revenue growth by personalizing its customer interactions.
In addition to increased revenue, there are many other ways banks can benefit from personalization:
• higher engagement and conversion rates
• stronger return on investment (ROI)
• improved customer loyalty and retention
• better customer experience
• consistent communication across all channels, etc.
When it comes to making the customer experience more personal, there are no tricks, it’s all about data and the ability to use it properly. Want to join the personalization trend and make the most of it? Explore our comprehensive guide to find out how recommendation engines help banks add a personal touch to their communication with customers, how to leverage data for more personalized CX, and how to efficiently scale your digital personalization efforts.
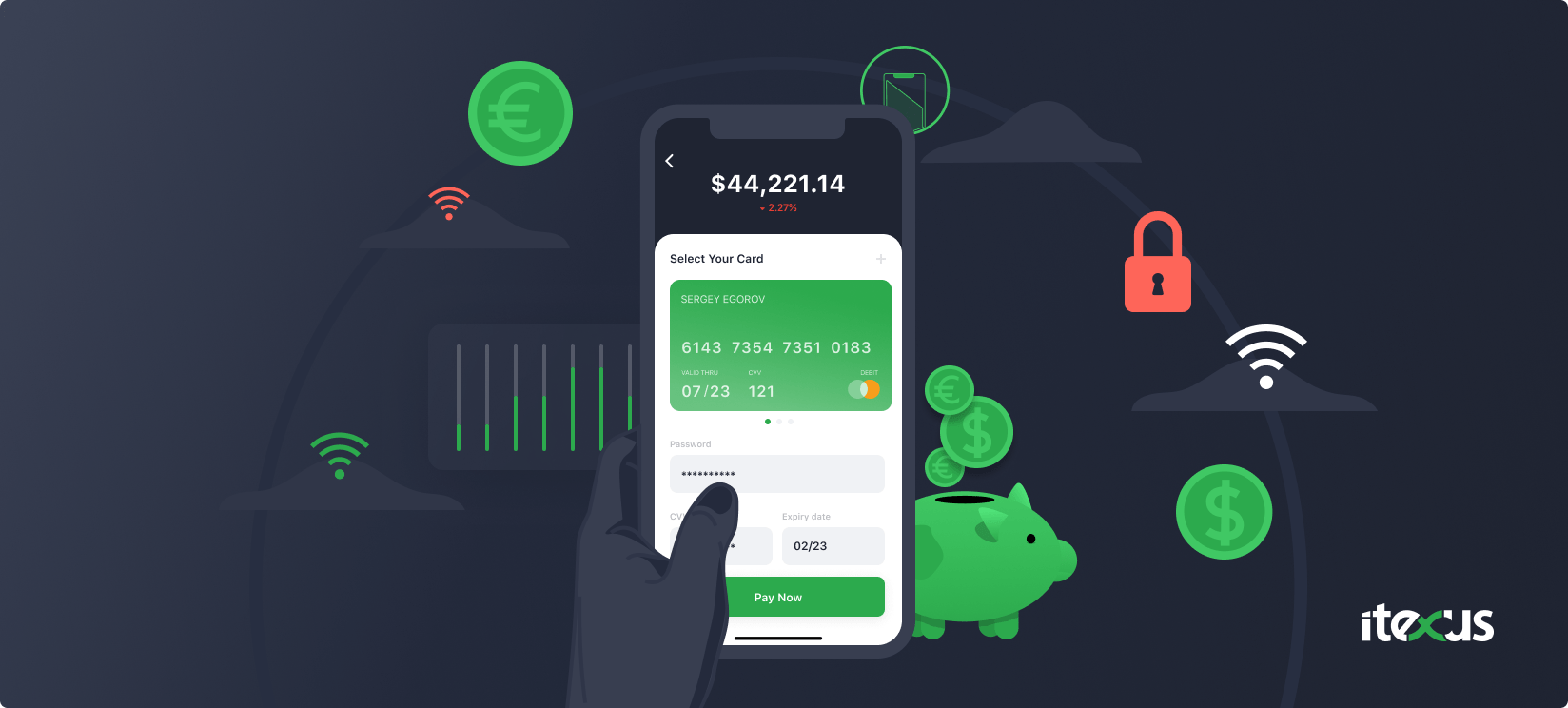
Personalized Banking Experience
In banking, personalization means delivering the right individual experience through the right channel at the right time. To do this, banks need a truly dynamic, decision-based marketing tool to target customers with the best and most timely offers in real time. Being fully integrated with their transactional and customer data, such a tool drives operational efficiency and increases revenue and customer engagement.
A recommendation engine can be one such tool. Let’s take a closer look at some of the processes you can transform with a recommendation system to make them more customer-centric.
Cross-channel personalization
The idea behind cross-channel personalization, also known as omnichannel personalization, is to provide a consistent customer experience and service across multiple channels simultaneously. It is best to combine digital channels with those that include human touchpoints. This method ensures continuous connectivity and helps create tailored customer journeys.
However, banks need to coordinate their efforts across channels and customer relationship management teams to get the most out of this method, as omnichannel banking does not break down silos in customer engagement and a lack of coordination can lead to customers getting lost in the gaps between unaligned channels. In such cases, a single recommendation engine helps streamline communication between bank departments and ensures that everyone is clear on the company’s CX strategy.
? Example of cross-channel personalization: a bank can track customer clicks across the site, measure the time spent on particular subjects and connect this data to analytics systems. Messaging can then be personalized based on this data. In this particular case, the customer’s online behavior may be a clear signal of interest in a particular product or service. Based on these signals, the bank can quickly make the right offer.
Personalized content
Creating data-driven content is an essential part of your personalization strategy. The content you design should reflect what the consumer wants to see and answer their questions. However, there is no one-size-fits-all solution, even though some content may seem to appeal to a broad demographic.
To tailor content to customers’ needs and goals, we recommend creating buyer personas based on your research data. You can adjust and optimize your content according to the needs of these small segments you have defined as your personas, and then advertise that content to the customers who are most interested in it.
? Example of personalized content: when it comes to onsite content, you can display personalized lead capture forms and pop-ups based on an audience segment. It could be banking product suggestions tailored to a customer’s needs – a better credit card based on spending, a lower mortgage APR based on current rates, etc.
Segmenting within the buyer’s journey
Basically, the buyer’s journey can be divided into four stages – awareness, consideration, decision, and assessment. The first-time visitor differs from a customer who is researching, and the latter differs from a long-term customer. Depending on where a customer is in the buyer journey, you can optimize your CTAs and content to encourage action. Note that a recommendation given to the customer at the wrong time, will show no response from them so this point should be considered seriously.
? Example of personalization depending on the customer’s place in the buying cycle: you can show personalized offers to returning website visitors based on their browsing history. If they have been browsing for mortgage loans, when they return to the site, the entire homepage should be personalized to highlight the most appropriate mortgage loan offers.
Recommendation Engine for Banking Personalization
At this point, you’ve should have a firm grasp of personalization and its role in the banking experience. Now let’s find out what technical means can help banks make the experience they deliver to customers more individual.
At the core of personalization is the recommendation engine. This is a type of data filtering tool that uses machine learning algorithms to recommend the most relevant products or offers for a bank’s customers. It collects customer behavior data and finds patterns in it.
Recommendation engines are ubiquitous: video streaming services like Netflix or Google use recommendation engines to suggest movies and series to viewers, online marketplaces use recommendation engines to provide product recommendations to customers, etc. The purposes of using a recommendation engine are slightly different, but the goal is the same: to drive sales, boost engagement, increase customer loyalty, and provide customers with a personalized experience.
Three main types of recommender systems
Collaborative filtering
A collaborative filtering engine collects and analyzes data about users’ behavior, activities, and preferences to predict what a person will like based on their similarity to other users. To make recommendations, a collaborative filtering engine uses a matrix-style formula. Such a system doesn’t analyze the content – it simply selects pieces to recommend based on what it knows about the user.
Content-based filtering
A content-based filtering engine makes recommendations based on a profile of the customer’s preferences and a description of an item they liked (genre, product type, color, word length, etc.), and uses this data to determine item similarity. Such a system is limited to recommending products or content that are similar to what the person already uses, and cannot go beyond that to recommend other types of products or content.
Hybrid model
A hybrid recommendation engine considers both collaborative and content-based data. Such a system generates natural language processing tags for each product or item and uses vector equations to calculate product similarity. A collaborative filtering matrix can then be used to recommend items to users based on their behavior, activities, and preferences.
How Does a Recommendation Engine Work?
A recommendation engine uses a combination of data and machine learning technology. Data is crucial to a recommendation engine because it is used to derive patterns – the more data an engine has, the more efficiently it will be able to make relevant suggestions. Recommendation engines go through a four-step process:
- Data collection
The first step is to collect data. There are two main types of data that need to be collected: implicit and explicit. Implicit data includes information from web search history, clicks, shopping cart events, search logs, etc. Explicit data is collected from customer input – this can be their likes and dislikes, product comments, reviews, ratings, etc. Recommendation engines also use customer attribute data to identify similar customers and feature data to identify product similarity.
- Data storage
The collected data needs to be stored. The amount of data will grow gradually, so scalable storage is required. Depending on the type of data collected, different types of storage can be used.
- Data analysis
In order to be used, the data must be analyzed. There are three ways to analyze data: real-time analysis (data is processed as soon as it is created), batch analysis (data is processed on a regular basis), and near-real-time analysis (data is processed in minutes rather than seconds if it is not needed immediately).
- Data filtering
Finally, the data must be filtered. For this, different mathematical rules and formulas are applied to the data depending on the type of filtering being used (collaborative, content-based, or hybrid). The result of this filtering is the recommendations.
Itexus Expertise in Recommender Engines Development
Building a good recommendation engine requires deep fintech expertise, first-class engineers, and robust development processes – all of which you get at Itexus. We have been delivering custom fintech solutions for our clients since 2013, and we are ready to develop an advanced recommendation engine for you, too.
Digital Insurance Marketplace with Recommender Engine
We developed a digital insurance marketplace with a recommendation engine for a Swiss consulting company with more than 20 years of experience in the insurance industry. The idea behind the project was to launch a new SaaS digital insurance marketplace that would fully automate the business processes for insurance companies and brokers, and provide end customers with a fully digital experience that allows them to select and buy an insurance policy online from multiple insurance companies and automatically recommends them the most suitable insurance plan. For more details on this project, see the original case study.
AI-Powered Financial Analysis and Recommendation System
Another recommendation solution we delivered was an AI-powered financial analysis and recommendation system for a fintech startup. The system uses machine learning techniques to process various content feeds in real time and increase the productivity of a financial analyst or an account manager in such domains as wealth management, commercial banking, and fund distribution. For more details on this project, see the original case study.
Summary
When a recommendation engine is built and set up correctly, it can bring multiple benefits to a bank:
• higher CR and ROI
• improved customer experience
• increase in upsell and cross-sell revenue
• reduction in customer churn
Personalization in banking is not a passing trend, so now is the time for banks to transform the way they interact with their customers by delivering more personal experiences through the right channel at the right time. At the moment, using a recommendation engine is the most efficient way for banks to scale their digital personalization efforts and to keep up with the increasing demands of their customers who expect to be treated individually. Let’s reshape your customers’ banking experience – contact us to find out how you can benefit from implementing a recommendation engine into the financial services your company offers.